On Marketers’ Minds: How Will Generative AI Affect my Marketing Operations?
Senior Technical Consultant
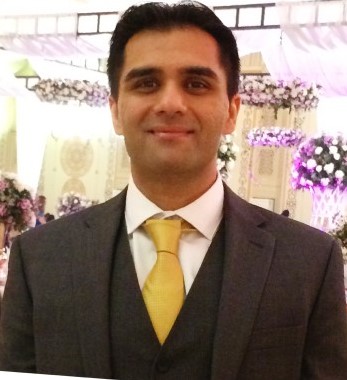
Ali Badshah
Client
Technology
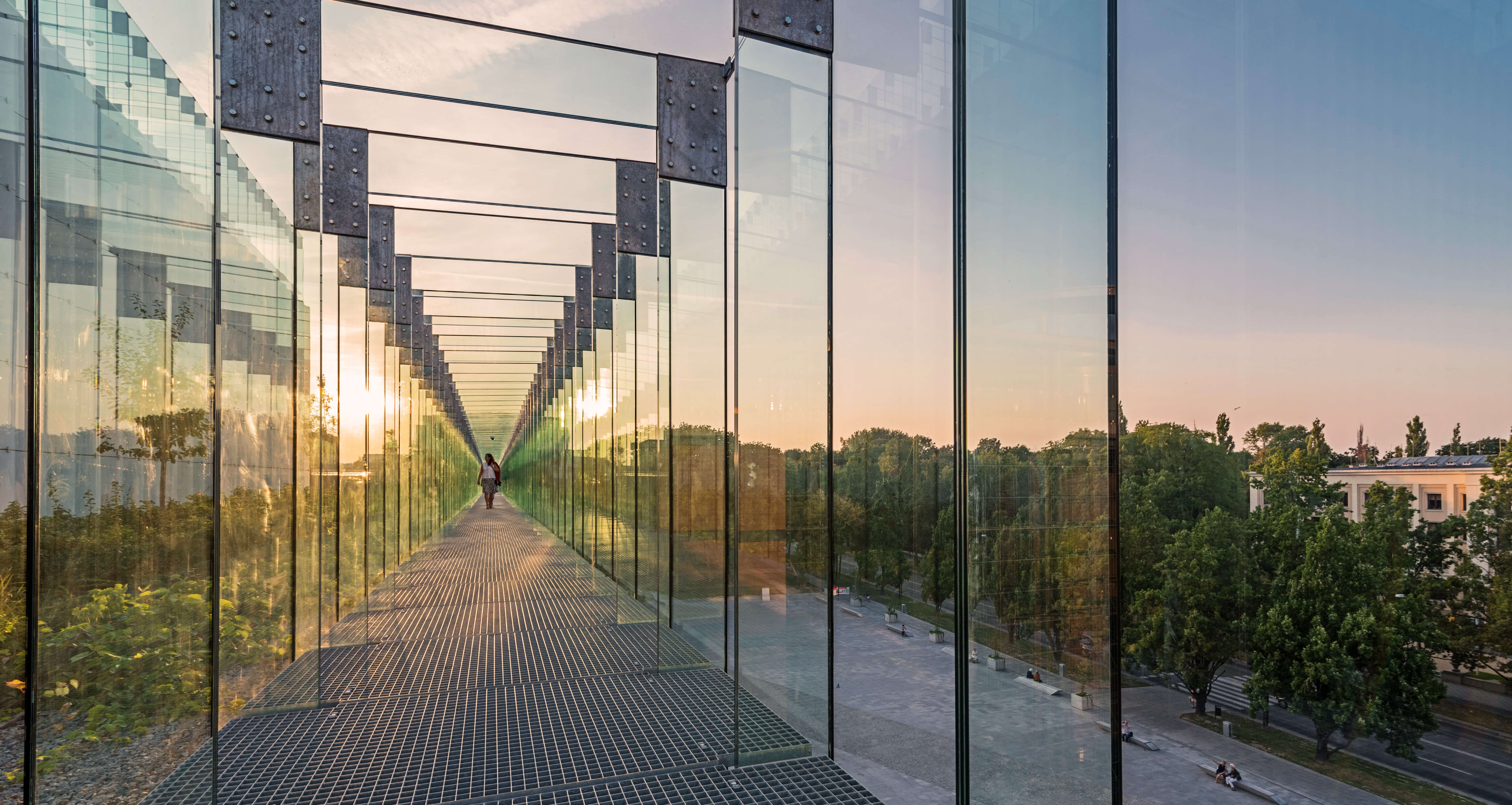
There’s a lot of interest and excitement surrounding generative AI these days. Sparked by the introduction of ChatGPT in November 2022, industry leaders and in-the-trenches practitioners are forecasting the impact of generative AI on everything from the future of work to how it can help kids do their homework.
Drawing from our expertise in marketing operations, technology platforms and the data that drives them, here are some of the big ideas, predictions and questions we believe every digital marketer should be thinking about when it comes to generative AI.
Changing Channel Inputs, Logic and Results
Generative AI has the potential to change the way search engines generate, display and prioritize results. Historically, consumers and brands have relied heavily on search as the starting point for shopping experiences. Over 80% of shopping experiences start with search[1] and, 50%+ of today’s digital advertising spend is allocated to support and drive this behavior.
Search and search measurement rely on keyword-based inputs that are combined with user-based signals. These signals drive the algorithms and auctions that return search results in a specific order. It’s easy to imagine a scenario in which keywords are exchanged for human-centric language requests – for example, through ChatGPT embedded in Bing or the capabilities of Google’s Bard – changing traditional search logic and bidding, the flow of results and the measurement and processes used to optimize performance.
Operations
We’ve seen many examples of generative AI’s ability to automate manual and mundane tasks. From writing a brief to building audience profiles and lists of exclusion URLs, generative AI has shown potential for efficiency gains and cost savings.
When it comes to more advanced and complex operations, generative AI could have an even broader impact. Search engines could use generative AI to match consumers to content and advertisers, transforming the way that campaign measurement, attribution and decisioning are done today.
For example, measurement and attribution would need to engage AI to predict how natural language strings affect future investment. Given the learning nature of generative AI, the value of a lookback window would be greatly reduced, and future predictions may only be valid for the next hour or until the next bid. In addition, depending on the channel and media, real-time attribution and AI-driven decisioning could drive a greater share of budgeting and bidding.
Going a little deeper…
Bidding & Spend: With the rise of generative AI, search that’s driven by natural language algorithms could shift away from keyword bidding to a more organized text combination, which forms the basis of language generation and processing.
As the algorithm learns, we could expect that the planning and bidding process would need to learn as well, which would affect the allocation of spend and, more importantly, the consumer experience. Though the future of AI-infused search and subsequent changes to buying and bidding (i.e. from keyword-based to “sentence and sentiment”) are still to be seen, marketers can and should begin planning and experimenting with this approach now.
Tagging and Tracking: Today, search plays a significant role in determining results that are driven by meta tags, code to text ratio, alt tags and text relevancy. Most search bots look for metadata on a page to identify and index content, which is later displayed on the search engines’ results page.
A shift from keyword combinations to general natural language requests raises a number of important questions:
- How will website rankings change, given traditional SEO strategies?
- Will information-rich, language-based meta tags need to be added to web pages so the AI can better understand content relevancy and return the “best” page based on the inquiry?
- Will content need additional definition in alt tags to support a language processor in evaluating and incorporating more image-dense pages into the recommended results?
Added requirements for tagging will drive modifications in tag, tag management, measurement, platform automation and workflow automation. Recent announcements by some of the big platform players, including Google, Microsoft and Amazon, speak of an increased role of generative AI in platform and cloud capabilities, but how that will affect cross-channel experience, signal capture and measurement is still yet to be seen.
Measurement & Attribution: In a world of generative AI, measurement and attribution will need to engage the AI “engine” to support decisioning – both human- and machine-based. Signal capture, processing, modeling and visualization must evolve to a more “always on, always optimizing” operating rhythm.
Data-Driven Attribution (DDA) will be a must-have in order for marketers and platforms to anticipate and target an audience with the content, messaging, sequence and frequency that will capture attention, drive engagement and a personalized experience. For marketers that aren’t already running DDA and/or Regression-Based Attribution (RBA) experiments, now is the time to start.
What’s Next
The world of generative AI is still a fluid space, producing more questions than answers. But our experience shows that a strategic framework for analysis and a culture of experimentation will enable you to understand and get ahead of some of the more probable shifts – minimizing risk and maximizing the benefit to your digital marketing and media operations.
In our next post, we’ll drill down into additional developments in generative AI, offering specific recommendations for how brands and advertisers can position themselves to take advantage of them now.
[1] Source: GE Capital Retail Bank, 2022 “81% of retail shoppers conduct online research before buying. The overwhelming majority of retail consumers start their journey with online research”.